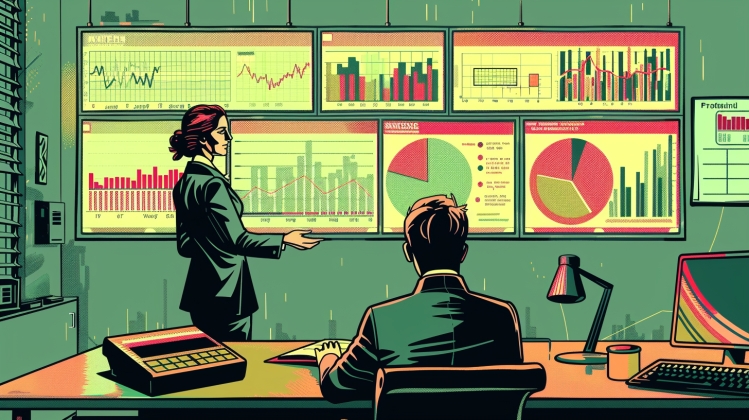
Data dashboards have become an ubiquitous component of modern business, and many organizations are racing to create custom tools or bundle them with other cloud-based services and data offerings. But that hasn’t stopped one team of entrepreneurs from entering the market with an eye toward reinvention: turning what some may perceive as just another status indicator into an efficient collaboration platform.
Writ, a San Francisco startup founded last month and announced today to VentureBeat its $3.8 million seed financing round led by Google Gradient Ventures with participation from Defy.vc, High Alpha Capital Management LLC (HAMC), Toba Capital LLC and other angel investors.
Adam Weinstein (CEO) and Jason McGhee (CTO) founded Writ in 2019. Cursor, their previous venture acquired by DataRobot in 2019, served as its predecessor solution.
At its core, Writ’s vision is simple: creating a dashboard designed for an AI-enhanced era that addresses all departments within an organization – not only data scientists or leadership. With it comes an auditable record of decisions taken with data by an organization and how well those outcomes turned out.
“For years now, companies have relied heavily on dashboards as one-way communication tools,” stated Weinstein in an interview with VentureBeat. He further commented on this issue and how companies could bring data and business teams closer together in providing offerings that go beyond mere data visualization to enable decisions based on it.
The company and its investors believe they have found the solution and that their custom dashboard tool can be leveraged by existing data scientists and team leaders, using AI, to provide more insightful graphics on-demand, faster, automatically across departments/teams, as well as providing an audit trail of any actions taken with data.
Weinstein stated, “Teams of people tasked with understanding a particular area of data such as sales or marketing operations teams or finance departments often lack tools that allow them to discuss and take the necessary actions,” adding:
Experienced founders
Both founders bring an impressive set of experience from companies like LinkedIn, Pandora, ExactTarget (now Salesforce), and Deloitte; this ensures they possess an in-depth understanding of data’s role in modern business practices.
Weinstein described how at one previous job he would keep partners informed on how their data access from parent company was being utilized. For instance, he would take screenshots from dashboards, “plop them into an email”, and send those around as updates.
Although this was useful for keeping partners informed, it failed to provide a good way of monitoring how this information was being utilized and keeping track of when decisions had been taken.
Weinstein noted, “there will likely be multiple emails and discussions to address any change or something of interest, with subsequent things beginning shortly thereafter; so if anyone wanted to delve further back and determine what happened, searching through their inbox would likely suffice.”
Writ’s goal is to understand how data has been utilized within an organization by different roles and departments in the past.
“What can you do there?” McGhee asked. “What can analysts do when they have monitoring in place?”
Already, Writ is working closely with partners and thought leaders to fine-tune the core functionalities of its product.
Although Writ is banking its success on using large language models (LLMs) and generative AI to power its intelligent dashboard products, rather than creating their own models from scratch, at this stage they prefer using leading off-the-shelf models from leading providers — noting their competition is fierce with new models coming out every week from competing providers.
“We don’t plan on investing a large sum in compute to try and create some “wonderful model,” McGhee told VentureBeat. Instead, their primary focus will be UX design partner needs and requests as well as understanding what exactly each partner wants from us.”
But Writ’s founding team recognizes the need to protect data privacy and security, so will not pass customer data onto third-parties nor train on it. Instead, when necessary they will use “metadata” and representational data instead, with local or virtual private LLMs only used when passing customer data to outside parties.