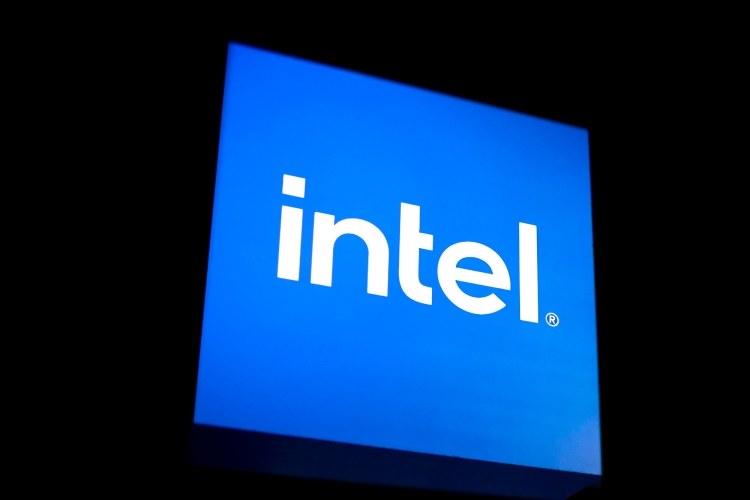
Intel Labs has taken an ambitious step toward developing computer systems capable of being as creative and adaptable as humans while operating more efficiently with less power consumption. Neuromorphic computing offers such promise – according to one blog post about neuromorphic computing it employs algorithmic approaches that mimic how our own brain interacts with its environment in order to deliver capabilities closer to human cognition and create capabilities closer to cognitive enhancement.
Intel’s neuromorphic computing research may seem futuristic, but its applications have already found practical use cases: adding voice interaction commands to Mercedes-Benz vehicles; designing robotic hands that deliver medication directly to patients or designing chips to recognize hazardous chemicals are just three.
Machine learning-driven systems such as autonomous cars, robotics, drones and other self-sufficient technologies rely on ever-smaller yet more-powerful and energy-efficient processing chips for their processing needs. But traditional semiconductors have now reached their miniaturization and power capacity limits, prompting experts to advocate a new approach to semiconductor design.
Gartner predicts that traditional computing technologies built around legacy semiconductor architecture will reach their digital wall by 2025 and necessitate changes, forcing companies like tech firms to adopt alternative paradigms like neuromorphic computing that mimics human brain and nervous system function via spiking neural networks (SNNs) where individual electronic neurons use spikes from individual electronic neurons to activate other neurons cascading along a chain.
Neuromorphic computing will enable rapid vision and motion planning at low power consumption, according to Yulia Sandamirskaya of Intel Labs in Munich. “These are key bottlenecks in creating safe and agile robots capable of targeting objects in dynamic real-world environments.”
Neuromorphic computing “expands the space of neural network-based algorithms,” she noted. By co-locating memory and compute in one chip, this allows energy-efficient processing of signals as well as continual, lifelong learning on one platform.
As AI computing becomes ever more sophisticated, a one-size-fits-all approach cannot satisfy every environment across its spectrum of application.
Neuromorphic computing could offer an attractive alternative to traditional AI accelerators by drastically improving power and data efficiency for more complex AI use cases ranging from data centers to extreme edge applications,” Sandamirskaya stated.
Neuromorphic computing resembles how the brain transmits and receives signals from biological neurons to identify movements or sensations within our bodies, yet unlike traditional approaches which organize computation in binary terms, neuromorphic chips offer more flexible computing power compared to their binary-oriented predecessors. Furthermore, their neural networks emulate natural learning processes by constantly remapping them allowing neuromorphic architectures to make decisions based on learned patterns over time.
These event-driven SNNs allow neuromorphic computers to achieve orders of magnitude performance advantages over conventional designs. Sandamirskaya pointed out that neuromorphic computing will prove particularly helpful for applications which must work under tight power and latency restrictions while simultaneously adapting real time to unpredictable conditions.
Emergen Research forecasts that neuromorphic processing industry will reach $11.29 billion globally by 2027.