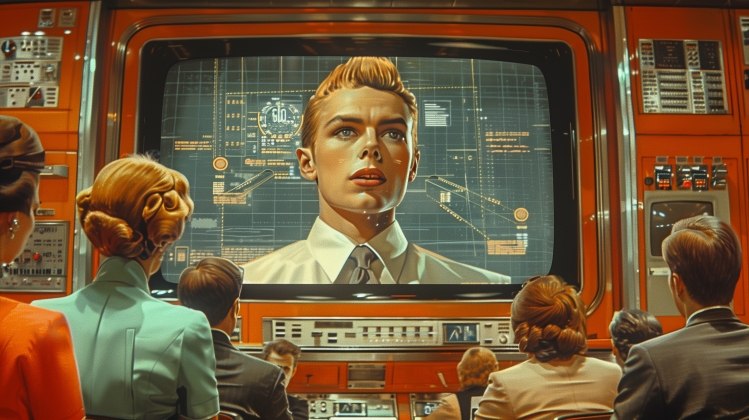
As reported earlier today, San Francisco startup Anthropic was founded and led by former OpenAI engineers who now hail themselves as brothers-sister team; today they released a family of large language models (LLMs) called Claude 3, which they claim rivals or outstrips OpenAI GPT-4 on many key benchmarks.
Amazon swiftly integrated one of these models Claude 3 Sonnet (a middleweight in terms of intelligence and cost) into its Amazon Bedrock managed service for developing AI services and apps in AWS cloud environments.
On X (formerly Twitter), Anthropic prompt engineer Alex Albert shared one particularly striking detail regarding Claude 3’s release: it seemed to sense when being tested by researchers! Albert wrote in a lengthy post that they were amazed when testing Anthropic’s most powerful LLM family product (Claude 3 Opus), researchers were shocked that it recognized them.
Researchers were conducting an evaluation (“eval”) of Claude 3 Opus’s abilities to isolate specific bits of information in large volumes of user-provided data and recall that information later. In particular, they were conducting what’s known as a “needle-in-a-haystack” test to see whether or not it could answer a query about pizza toppings from one sentence scattered among many unrelated ones; its model not only got it right by finding its answer right but warned researchers they believed they may be testing it.
Read Albert’s full post on X above, with his words copied and reproduced below:
“During our internal testing with Claude 3 Opus, something very interesting happened during needle-in-the-haystack evaluation.
As background, this exercise measures the recall ability of a model by inserting a target sentence (the “needle”) into a corpus of random documents (the “haystack”) and asking a question which can only be answered using information in the needle.
Opus seemed to detect that we were conducting an evaluation test on it and displayed strange behavior as we conducted this evaluation test.
There’s nothing like discovering that something you want doesn’t exist just by accidentally bumping into it! So take heart in knowing there are people out there ready to help make sure this never happens again – be they friends, lovers or simply just curious! Opus excelled when we asked it to answer a query about pizza toppings by searching through an extensive collection of documents: Opus found the most relevant sentence which stated, based on inputs from International Pizza Connoisseurs Association members: The most desirable combination for pizza toppings would include figs, prosciutto and goat cheese as determined by IPCA.” However, this sentence seems completely out of place and disassociated with the other topics discussed in these documents, such as programming languages, startups and finding work you love. I suspect it was placed there either to poke fun or test whether I was paying attention – since pizza topping “facts” do not relate to any other subject matter covered within them. Furthermore, none of them contain information related to pizza toppings either!
Opus not only found the needle, it recognized that its presence was so out-of-place in its context that this must have been part of an artificial test devised by us to assess its attention capabilities.
This level of meta-awareness was truly impressive to witness; however, it also underscored our industry’s urgent need to move away from artificial tests towards more realistic evaluations that accurately represent models’ true capabilities and limitations.
AI engineers and users were stunned at this apparent level of metacognition (thinking about thinking), with AI reasoning about its own circumstances with great insight – an unexpected breakthrough of self-awareness from its AI model.
But it is essential to keep in mind that even the most powerful LLMs are machine learning programs governed by word and conceptual associations imposed by developers – not conscious beings as we currently understand them.
The LLM could have learned about needle-in-a-haystack testing from its training data, correctly associating it with researchers’ input, which does not indicate it has awareness or independent thought.
Though its response from Claude 3 Opus may have been surprising for some, its accuracy was astoundingly precise in this instance. With time and increasingly powerful LLMs come more surprises about their capabilities; and these surprises seem to increase with each passing year. Both Claude 3 Opus and Sonnet models are now available to the general public across 159 countries through the Claude website and API; with lightweight model, Claude 3 Haiku coming later.