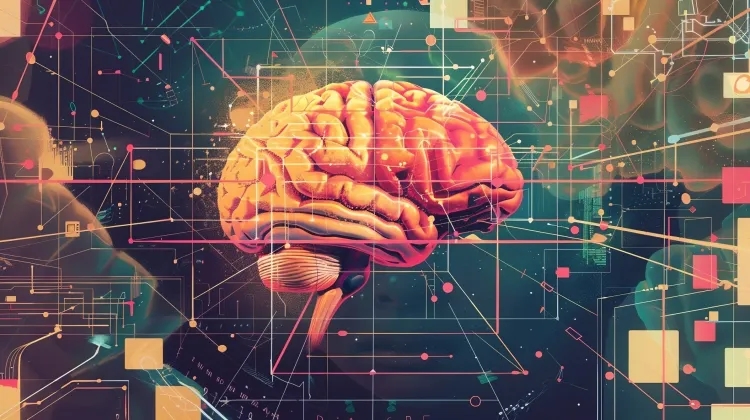
Artificial intelligence startup Symbolica unveiled today its innovative approach to AI modeling construction: using advanced mathematics to imbue systems with human-like reasoning abilities and unparalleled transparency. Their aim is to move away from current AI’s “alchemy” towards more rigorous, scientific foundations.
In conjunction with its public launch, Symbolica announced today it has raised $33 million in total funding (Series A + seed) led by Khosla Ventures with contributions from Day One Ventures, General Catalyst, Abstract Ventures and Buckley Ventures.
“Symbolica’s founder and CEO George Morgan recently told VentureBeat: ‘We aren’t building models; rather, our focus lies in developing an architecture generation process which is far more powerful than anything previously possible’.”
Morgan was previously employed at Tesla as a senior autopilot engineer and worked on its self-driving systems. Following this experience, he founded Symbolica with a team of Ph.D. mathematicians, machine learning (ML) researchers, engineers from Tesla, Neuralink and ClearML as well as world renowned creator of WolframAlpha and Mathematica Stephen Wolfram and American Mathematical Society Fellow Stephen Wolfram as advisors.
At Symbolica’s core is “category theory”, an approach to mathematics which formalizes mathematical structures and their relationships. By grounding AI within this rigorous framework, they believe they can create models with reasoning capabilities as an inherent attribute rather than simply as an outcome of training on massive datasets.
Morgan likened modern AI systems, particularly deep learning ones, to alchemy before modern chemistry was created. “AI architectures lack any form of scientific oversight today – no other discipline of engineering does!” said Morgan.
He used an analogy between drug discovery and AI or machine learning: Imagine trying to invent Tylenol: you wouldn’t simply combine various substances together in hopes that something magical happens; instead, you would consider how chemicals bind with specific receptors, the interactions among molecular structures in relation to those receptors, etc. In other words, there would likely be an established scientific process associated with solving such a challenge which doesn’t currently exist in AI/ML systems.”
He contended that lack of rigor makes current AI models opaque black boxes; once trained, one has no idea of the internal mechanisms and structures it has learned or how it has formed associations.
Opening the Black Box
Symbolica’s approach aims to open up that black box, providing interpretability. “By describing an architecture we can also describe its learning behaviors; what types of structures it may learn or embed inside that architecture. And this provides one pathway towards interpretable AI models,” Morgan noted.
Interpretability is becoming ever more essential as AI becomes integrated into more high-stakes decisions across industries such as healthcare and finance, while regulation remains key for AI systems. “Symbolica gives an extremely formal, precise definition for understanding models,” Morgan noted, and this can help regulate models effectively.
Symbolica’s approach offers AI systems capable of performing complex reasoning tasks with far less training data and computing power than existing models, according to Morgan. Building architecture capable of native reasoning takes far fewer data inputs to get that model performing as effectively as unstructured models that lack such functionality, according to Morgan.